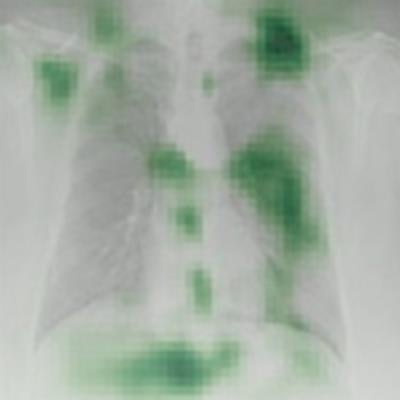
Researchers have developed an AI model that can accurately identify patients with type II diabetes from routine frontal chest x-rays, according to a study published July 7 in Nature Communications.
The deep-learning (DL) model combines features on ambulatory chest x-rays and electronic health record (EHR) data and flagged 1,381 cases (14%) as suspicious for type II diabetes in a test dataset of 9,943 chest x-rays, the group found.
"These findings demonstrate the potential of DL models in harnessing demographic and administrative EHR data for disease discovery," wrote corresponding author Ayis Pyrros, MD, of Duly Health and Care, and colleagues.
Chest x-rays are not the primary method for detecting type II diabetes, yet they can be used to measure biomarkers associated with the disease. This includes visceral fat and body mass index (BMI), which are independent risk factors for the disease, the researchers explained. Moreover, while diabetes rates are increasing, detection rates have remained level since the 1980s.
Thus, the group hypothesized that a DL model -- specifically one trained to generate visual findings (so-called "explainable AI") -- could help narrow this gap.
The researchers trained the DL model to predict type II diabetes using 271,065 ambulatory chest x-rays from 160,244 patients. As well as labeled images, input data included information from ICD9 and ICD10 codes used in EHR claims processing, which include diagnoses and descriptions of symptoms. Additional training data included each patient's body mass index (BMI).
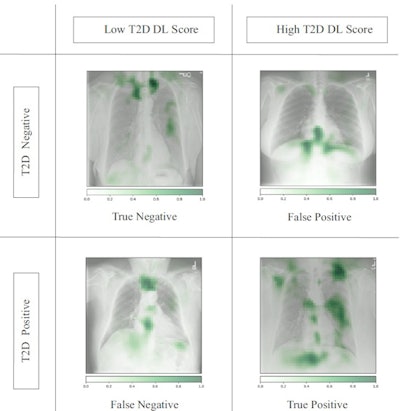
The prospective test dataset of 9,943 images included 8,382 (84.3%) patients with no type II diabetes, 1,119 (11.3%) who had controlled disease, and 442 (4.4%) with poorly controlled disease. According to the findings, the model effectively detected type II diabetes with an area under the receiver operating curve (AUC) of 0.84 and a 16% prevalence. The model flagged 1,381 cases (14%) as suspicious for type II diabetes.
Finally, the authors noted that the occlusion maps generated to display the basis for the DL model's decisions corresponded significantly to known fat distribution features for the disease in the central chest, lower neck, upper abdomen, and axillary regions.
"We believe the detection of this central mediastinal adiposity is why the DL model is able to predict [type II diabetes] in patients," the researchers wrote.
Ultimately, because chest x-rays are the most common imaging exam in the world, such AI models could be applied to large populations to identify high-risk individuals and perform more accurate risk assessment, the researchers concluded.
Click here to read the full study.