Abstract
In low- and middle-income countries (LMICs), AI has been promoted as a potential means of strengthening healthcare systems by a growing number of publications. We aimed to evaluate the scope and nature of AI technologies in the specific context of LMICs. In this systematic scoping review, we used a broad variety of AI and healthcare search terms. Our literature search included records published between 1st January 2009 and 30th September 2021 from the Scopus, EMBASE, MEDLINE, Global Health and APA PsycInfo databases, and grey literature from a Google Scholar search. We included studies that reported a quantitative and/or qualitative evaluation of a real-world application of AI in an LMIC health context. A total of 10 references evaluating the application of AI in an LMIC were included. Applications varied widely, including: clinical decision support systems, treatment planning and triage assistants and health chatbots. Only half of the papers reported which algorithms and datasets were used in order to train the AI. A number of challenges of using AI tools were reported, including issues with reliability, mixed impacts on workflows, poor user friendliness and lack of adeptness with local contexts. Many barriers exists that prevent the successful development and adoption of well-performing, context-specific AI tools, such as limited data availability, trust and evidence of cost-effectiveness in LMICs. Additional evaluations of the use of AI in healthcare in LMICs are needed in order to identify their effectiveness and reliability in real-world settings and to generate understanding for best practices for future implementations.
Similar content being viewed by others
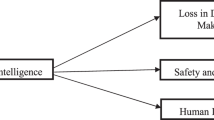
Introduction
Rapid technological developments of the past few decades have paved the way for an abundance of technologies that have and continue to revolutionise medicine and healthcare1,2,3. The field of artificial intelligence (AI), in particular, benefits largely from the expanding accessibility of the internet, the progression in software system development, and the fast advancement of microprocessor technology that translated into a variety of widely available devices including tablets, smartphones, laptops and virtual reality appliances4. With a widely recognised and accepted definition still underway5, this paper uses the definition by Russel and Norvig which describes AI as the wider field of “designing and building intelligent agents that receive precepts from the environment and take actions that affect that environment”6.
Particularly relevant AI technologies in medicine and healthcare include knowledge engineering, machine learning (e.g. precision medicine, neural network models), natural language processing, rule-based expert systems, surgical robots, or image and signal processing7. Medical education, clinical practice and healthcare delivery have all benefited from these technology advancements, which have offered new techniques and methodological approaches. AI is revolutionising the foundations of healthcare with its potential to improve both the scope and accessibility of healthcare provision at a global scale8,9.
Given these technological developments, AI has the potential to substantially change the role of how medical care and public health programmes might be implemented in the near future, especially in health systems where the distributions of and access to care have so far been challenging3,10. In low- and middle-income countries (LMICs), the value of AI is seen in its potential to build health systems by supporting and standardising clinical judgement and applying healthcare processes more objectively with a data-oriented approach11. Furthermore, given the shortages of skilled health workers in areas such as sub-Saharan Africa, where medical education capacities are limited12, AI-powered clinical tools could represent one way to increase quantity and quality of medical care13. However, current AI applications and machine learning still require large amounts of complete and regularly updated datasets, which still remain scarce for most LMICs14. While reports on the application of different AI technologies in LMICs continue to grow, the actual evidence base has so far not been reviewed. The scope and extent of implemented AI remains unclear, or whether AI technologies have proven to have potential for healthcare delivery in LMICs.
The goal of this systematic scoping review is therefore to review and map existing literature on health-specific AI applications and to summarise currently available evidence of AI’s efficacy across LMICs. To allow for a comprehensive outline of AI technologies applied to both medical practice and healthcare delivery, this paper systematically reviews and identifies all relevant literature across a wide range of AI applications, health systems, and LMICs. A further focus is on strengths, weaknesses and perceptions of the application of AI in healthcare in LMICs, exploring the following questions:
-
What are the effects of current AI-based technology on healthcare provision (e.g. diagnosis, treatment, health outcomes, provider or patient time, costs, etc.)?
-
What are the experiences of providers and patients with respect to the application of current AI-based healthcare technology (e.g. acceptance, perceived usefulness, trust in technology, feasibility to implement and integrate, etc.)?
-
What are key elements that support or challenge AI implementation in the LMIC healthcare context?
Results
Eligible records
Our database and handsearch identified a total of 1126 articles, of which 1104 were included in title and abstract review after removal of duplicates (see Fig. 1 for details). The final sample of peer-reviewed articles entering analysis included a total of ten studies, described in Table 1. A list of references for the included studies is available in Supplementary Note 2.
Study characteristics
Four studies were conducted in China, while the other six represent a range of LMICs across Latin America, South Asia and Sub-Saharan Africa (see Table 2 for a summary of key characteristics). Overall, a majority of studies (n = 8, 80%) were conducted in the context of upper-middle-income countries. All identified studies have been published since 2018 onwards. While most studies are based on cross-sectional designs, these varied in their quantitative and qualitative methodologies. Study populations ranged from 12 in a clinical research setting to 45,000 in research involving mHealth platforms15,16.
Features of studied AI technologies
Table 3 summarises the features of the studied AI technologies. Of the AI technologies studied in the reviewed articles, three were applied to the care of communicable diseases (two to HIV/tuberculosis, one to COVID-19), four to the care of non-communicable illnesses (three to various cancers, one to child nutrition), and three to general primary healthcare including pregnancy care. Within their clinical context, three technologies were applied to patient triage, four to screening or diagnostics, and three to care planning or provision. Of these, three tools assisted with triage and screening tasks performed by frontline health workers17,18,19. Four tools assisted physicians with diagnoses, clinical decision making and treatment planning15,20,21,22. Two articles studied the use of chatbots by individuals in the community, one being an ‘AI Doctor’ for primary care self-diagnosis23, and another offering social support messages on a health forum24. Two articles examined AI technologies used in distributing health educational information and support on child nutrition or pregnancy-related care with target populations in the community16,24.
Transparency of data and algorithms used in training AI tools
Overall, included studies varied regarding the extent to which datasets and algorithms used in the training and testing of AI tools were made transparent. Further, none of the datasets described in any of these studies were immediately accessible to the public in full. Five studies, however, provide reference to the datasets used15,16,17,18,24, and five studies described the AI algorithms used in detail15,16,17,21,24. Studies using commercially available products provided limited or no information on their respective datasets and algorithms18,19,20,22,23. Information gathered about the datasets and algorithms used can be found in Supplementary Table 2 and the Supplementary Discussion.
Interpretability of AI models
Most AI tools (n = 7, 70%) lacked any interpretability of their outputs, using ‘black-box’ algorithms15,16,17,21,22,23,24. A total of two AI tools for diagnosing TB or COVID-19 using chest X-rays provided interpretable heatmaps/areas-of-interest on a chest X-ray18,19. One study used IBM Watson for Oncology, a cancer treatment planning assistant, which provides relevant literature, such as clinical trial data, for a particular treatment it has recommended - though it is still largely a black-box tool20,25.
Strengths, weaknesses and perceptions of implemented AIs
In the next sections, we focus specifically on cost-savings and improvements in health outcomes, effect on workflows and time to treatment and diagnosis, local adequacy of AI, and user-friendliness, reliability and trust in AI technologies summarised in Table 4.
Reliability of AI tools
Concordance between the AI tools and physicians was reported in four studies17,20,21,22. Perfect concordance was reported in small samples of triaged breast lumps using ultrasound and radiation treatment plans17,21, but also some discordance between clinical decision support systems and the local treatment options available20,22.
Concordance of the IBM Watson for Oncology between physicians’ clinical decisions and treatment suggestions varied from 12% to 96% across several cancers20. This included cases where a suggested treatment was too expensive, not available, considered to be too aggressive or inconvenient for the patient, or locally available alternatives would have been preferred. Baidu Inc’s ‘Brilliant Doctor’ clinical decision support system made generally good suggestions, but sometimes disagreed with physicians on their first choice of diagnosis and treatment22. Participating physicians reported that inadequate care recommendations were usually a result of the system’s poor interoperability with other IT systems, use of inaccurate information, and missing information on patient’s income and insurance background22. The misalignment with the local clinical context was attributed to the training protocols used. For example, both tools were trained on data outside of their applied contexts, and thus did not fully account for the local disease incidence and treatment options available20,22.
Effect on workflows and time to treatment and diagnosis
AI technologies improved workflows in a number of ways. Non-sonographer healthcare workers (HCWs) could be trained in AI-based ultrasound triage, thus reducing the workloads of formally trained sonographers17. Similarly, automated radiation treatment planning reduced the time spent by radiation oncologists in making treatment plans21. COVID-19 triage was also more time-efficient in an Ecuadorian hospital once an AI computed tomography (CT)-screening tool was implemented18. The ‘Brilliant Doctor’ clinical decision support system also had a partially positive impact in rural Chinese primary-care clinics by suggesting diagnostic alternatives to physicians, thus facilitating medical information search and potentially reducing the likelihood of medical errors22. Notably, however, higher workloads were reported in clinical settings with low capacity for adopting new AI tools15,22.
Shortened time to diagnosis or treatment were reported in two studies. Delft’s ‘CAD4TB’ TB screening tool reduced time to the initiation of treatment compared to standard sputum screening tests in a Malawian primary-care clinic, while a social support chatbot improved response times for individuals seeking social support in online forums in China19,24.
User-friendliness and compatibility with existing infrastructure
User-friendliness and compatibility with existing infrastructure are quintessential in this context, as healthcare personnel or patients may not be trained or used to using new technologies while being short on time, resources and making potentially life-changing decisions under pressure. These aspects were noted in four of our included studies.
The ‘Brilliant Doctor’ clinical decision support system was found to require too much information from physicians, which was perceived as too time-consuming in a majority of cases22. Lacking integration with existing IT systems also resulted in critical laboratory information not being factored into the AI’s decision making process22. Physicians in Peruvian TB clinics also reported problems with an app-based TB-diagnostics tool utilising chest X-rays, including issues such as crashes of the app or mistranslations15. Poor internet connectivity inside the clinics and the overall limited availability of X-ray viewers throughout clinics impeded the uploading of X-ray images to the TB diagnostic tool by nurses15.
Fan et al.23 reported that self-diagnosis chatbots were used mostly by younger patients. Although a majority of user feedback for the ‘Doctor Bot’ chatbot was positive, some chatbot users also perceived the provided information to be insufficient, overwhelming, or difficult to understand23.
Garzon-Chavez et al.18 reported a rather successful incorporation of the chest CT AI-assisted triage tool into the hospital’s COVID-19 triage process which required cases identified as high COVID-19 risk arriving at the emergency room to first undergo CT-based screening. Later during the pandemic, once Reverse Transcription Polymerase Chain Reaction (RT-PCR) tests became more readily available, the AI-assisted chest CT scans remained the dominant form of triage due to its speed despite lower accuracy.
Trust in AI systems
User-friendliness is linked to another critical point when introducing AI systems in healthcare: end-user trust in the technologies. Two of our included studies discussed user trust in AI technologies.
Physicians interviewed in Wang et al.22 expressed distrust in clinical decision support systems, as the basis on which diagnostic or therapeutic decision-making occurred was not sufficiently transparent. Similarly, Fan et al.23 reported that diagnoses produced by the AI self-diagnosis chatbot were perceived as inaccurate by some users.
Wang et al.24 further pointed out problematic behaviour by their social support chatbot, whose identity was hidden from end-users on an online social support forum. In one case, in comforting a user who recently had a child, the AI mimicked a human response implying it had the same experience with its own baby. Given the chatbot’s identity was hidden, this raised questions about how AIs should be trained in order to avoid responding inappropriately to user posts24.
Cost-savings and improvements in health outcomes
Only MacPherson et al. conducted a cost-effectiveness study of their AI tool. Compared to usual care, the AI ‘CAD4TB’ TB-screening tool improved patients’ quality-adjusted life-years (QALYs) by reducing the average time to receive treatment. However, the cost per QALY was measured to be $4,620·47 per QALY gained, which was deemed to be beyond the willingness-to-pay in the Malawian context19. Wang et al.24 found that an AI chatbot performed comparably with humans in promoting positive responses from online forum users seeking emotional support.
Local adequacy of AI
Local adequacy of AI tools was a common theme in our studies, with three studies discussing challenges with applying AI tools to new lower-resource contexts.
Zhou et al.20 suggested the US-based training of IBM Watson for Oncology using US medical literature has led to inappropriate treatment suggestions in the Chinese context. Ugarte-Gil et al.15 reported unexpected complications with the implementation of their TB diagnostic tool, with their implementation sites having less internet connectivity, X-ray viewer capacity and mobile technology proficiency among health care workers than they had expected, which reduced the effectiveness of the AI tool. Wang et al.22 reported the AI clinical decision support tool had not well accounted for rural primary-care physician workflows in its design, and its usefulness could have been improved as a triage assistant rather than a physician assistant.
Discussion
The literature on AI applications for healthcare in LMICs has been steadily growing in recent years and is so far largely dominated by studies and reports from China and India26. Despite the substantial improvements in the technical capabilities of AI in different branches of medicine, such as ophthalmology and radiology27,28, many studies were not included in this review because they were proof of concepts and did not describe AI implementations in real-world, low-resource settings, limiting our understanding of the true performance and benefits of AIs29. This research is critical to understand both the adaptation to and potential performance of AI tools to medical and other health-related fields in settings where this technology has so far not yet played a strong role30. However, we found that researchers are actively addressing this knowledge gap. We came across a rather large number of LMIC-based publications of research protocols related to planned or on-going AI evaluations, as well as studies published since the time we performed our literature search that would have met our inclusion criteria. For instance, recent ophthalmology studies from Thailand and Rwanda have demonstrated the potential of AI-assisted diabetic retinopathy screening in LMICs while also flagging issues similar to those of our included studies, such as the challenge of integrating AI systems into existing workflows and infrastructure31,32. The private sector is also highly active in developing AI tools for healthcare, as our grey literature search revealed (see Table 5). None of the AI tools described in the grey literature provide concrete evidence to show that they improve health outcomes, or reduce costs associated with healthcare, although one can assume that some tools are hugely beneficial, such as automated drone deliveries of medical supplies in rural Rwanda33. Increased efforts to provide prospective evaluations of such tools would be beneficial for the wider healthcare community by offering lessons in which AI tools can improve health outcomes and/or reduce costs in particular contexts, and what may be required for said AI tools to be successful in their implementation.
The performance of AI applications in healthcare settings varies greatly, as was also observed in previous reviews of AI applications in medical imaging when compared to clinicians34,35. This is similar to included studies in this review that found inconsistencies in diagnostic sensitivity and specificity between AI tools and physician assessments17,18,20,22. Also, we were unable to identify many studies performing prospective feasibility testing or trials of AI tools in real-world settings in order to test their performance34,35. The reported performance of AI tools tested on retrospective datasets should be treated with caution, as a tool’s accuracy likely diminishes when applied to new data from different contexts18,35. Further studies of the performance of AI tools applied in healthcare settings are required to take into account data and concept drift36. Based on our review, existing evidence is also limited by inconsistent levels of reported transparency with respect to AI implementation and performance. For instance, there seems to be no systematic approach to report on the use of the type of datasets used for AI training, testing and validation, the underlying training algorithms, and key AI outputs that would allow a more direct comparison of AI performance, as well as to identify potential causes of poor performance34.
The underlying dataset is a key element of training the AI tool and its performance. Data from included research suggested that AI systems were trained on data collected outside of the implementation context17,20,22. However, AI-models trained on high-income country data may introduce bias into AI outputs, leading to poor performance or, worse, wrong results - which is harmful in a health-context and also harmful in establishing AI in healthcare because trust may be broken. Given that data is dynamic and may change its statistical features over time (data and concept drift), it is critical that AI models receive context-specific and updated data on a frequent basis; otherwise, AI models’ performance may worsen over time. This could lead to a downward spiral, as poor performance is likely to lead to poor acceptance of HCWs and a loss of trust in AI-based systems. While middle-income countries, like China and South Africa, have substantial collections of data pertaining to both the health system and health service delivery at the national and sub-national levels, the selection of training data is more limited in many low-income countries11. On the other hand, available context-specific data sets might be underused, untapped, or deemed too limited or inadequate, as the contained information is too asymmetric, asynchronous or varied in type, and spread across locations to facilitate reliable AI training11. There are no clear estimates on the amount of training data needed in designing an AI project. To better leverage small datasets in the context of LMICs, additional modelling techniques and simple classifiers should be considered, like the Naive Bayes algorithm, which allows a sufficiently strong learning process if applied to small datasets37. While public health institutions, donor-funded programmes and the business sector all generate large volumes of data, such data is often inaccessible to researchers and AI implementers38. Data collection and storage is too fragmented, or only intended for very specific purposes, such as programme reporting, policy development, strategic planning and advocacy39. Furthermore, some LMICs still face challenges in digitization of routinely collected data, as well as limited digital literacy with respect to data collection and management38. Ongoing efforts to harmonise fragmentations in health information systems that foster accurate, reliable, timely, interoperable datasets will be crucial in advancing AI technologies38. Routine data collecting platforms, such as OpenMRS or DHIS2, are well-established in low- and middle-income countries, and other initiatives, such as Health and Demographic Surveillance Systems40, provide enormous and standardised population health datasets encompassing decades. Yet, data ownership and data sharing rules can still pose barriers to accessing this data for research and commercial purposes. The Nairobi data sharing guidelines of 2014, as well as the Global Digital Health Index, are both first steps toward finding solutions to this topic. In order to develop datasets that may be used for AI, privacy regulations, data access and ownership agreements, and other essential challenges must be overcome. Public health agencies can play an important role in encouraging data sharing and providing public access to health data – both internal and private-sector generated health data – while also developing the governance mechanisms required to protect individual privacy.
Usability and integration of digital health tools, including AI tools, remain a challenge in high- and low-resource settings alike. Coiera41 and Cabitza et al.42 identified some of the complex challenges of the “last mile of implementation” that cause a poor translation of statistically high-performing AI into real-world applications. Especially in low-resource settings, the effectiveness of AI tools depends on how well these technologies can be utilised or integrated by end-users within an existing infrastructure43. In order to perform well in a real-world setting, AI tools should complement existing organisational networks of people, processes and technologies41,42. Inadequate design of user-interfaces can further limit the positive impact of an AI tool on clinical applicability, irrespective of diagnostic accuracy42. Complex or confusing user interfaces can lead to frustration among end-users or limited successful tool application, negatively impacting the uptake of technologies by front-line health workers or patients in low-resource settings15,22,44. Successful introduction of novel digital tools in low-resource settings therefore needs to account for and increase the basic capacity of HCWs to adopt technologically complex tools44. In some of the studies included in our review, AI integration was limited due to incompatibility with existing electronic health record systems, which in turn limited its performance as decisions could not be fully supported by relevant health record data. Another barrier to successful AI implementation includes the often unstable internet connectivity in some low-resource areas, since poor or intermittent internet access disrupts the use of cloud-based tools needed to upload key data elements, such as radiology images44.
Trust and acceptance of users is a critical feature of AI for global health and healthcare in general. Trust in AI applications has been found to be stronger if a technology and algorithms are understandable and assist users toward their goals45. A majority of reviewed studies still relied on a ‘black box’ approach, which leaves it unclear how the algorithms used eventually arrive at results. Furthermore, only half of studies provided a transparent description of their AI methodologies. Healthcare AI should be transparent about the source of data, qualify AI-based suggestions, and be explainable when they are used by clinicians and patients to make decisions46. Otherwise, it could negatively affect the trust foundations and increase the likelihood of rejection of the healthcare AI technology. Of course, patient data security is an essential aspect, particularly as cyberattacks get more sophisticated47. The adoption of approaches and structures similar to those regulating the pharma industry and the production of medicines might therefore be a feasible path forward for AI in healthcare. Likewise, AI healthcare applications may need to go through a similar process of preclinical research, clinical research, authority evaluation and post-market safety monitoring. It is also necessary to investigate future revisions of medical curricula to incorporate elements strengthening future HCW’s digital literacy and knowledge which may increase trust and effective usage of technologies, such as AI-based systems. Currently, users often have not received sufficient training and feel overwhelmed. Therefore, digital systems are often regarded as additional burdens. Another approach that appears to build user trust and hence potentially boost technology acceptance is the slow introduction of innovations, which “allows for incremental social learning”45. In general, technology acceptance is a complex process48. Other factors, such as a thorough understanding of the users’ benefits in contrast to other available technologies and pathways, undoubtedly play an essential part in lessening innovation resistance. It seems beneficial to proactively communicate from the start of the development process45. Overall, trust is a complex and delicate component and should be a key priority particularly at the start of the wider implementation of AI-based healthcare applications. Otherwise users, both patients and health care workers, may reject the technologies and impede further progress.
Affordability is an important characteristic of AI tools in a LMIC context. Even if the technologies are efficacious, this benefit cannot be realised if they are more expensive than legacy approaches to which HCWs are familiar. Our review and the wider literature suggest there is a dearth of evidence on the improvements in health outcomes and cost-savings associated with the implementation of AI tools in any context49, and of eHealth tools more generally50. We hypothesise that this finding reflects the maturity of AI healthcare research, since cost-effectiveness analyses necessarily occur later in the AI tool development and implementation timeline. To evaluate whether AI tools are affordable in LMICs, there is a need for more cost-effectiveness analysis studies.
A number of local challenges were reported in the studies included in this review as well as the wider literature. AI-based systems were not sufficiently integrated in existing workflows and infrastructure; healthcare centres in LMICs are subject to system outages caused by power or internet connectivity disruptions15,32,51, and, as a result of donor-funded agendas in LMICs, there is intermittent advancement that is susceptible to trends or “fashions”38, eroding faith in these systems further due to their lack of utility and continuity. Additionally, there seems to be a concern among HCWs in LMICs that AI may eventually take over their jobs, impeding its further adoption52 .AI applications in healthcare require a holistic systems approach to implementation. Consideration of the multiple interacting facilitators and barriers to AI implementation in real-world settings is required, in addition to the technical performance AI system in addressing a specific health problem, in order to have maximal impact on human health. Future implementations may also want to consider ‘effective coverage’ - the need, use and quality of health intervention - as a performance metric53. Further studies are required in order to address contextual challenges, such as trust and HCW job insecurity, data insecurity and sustainability, in order to inform future AI implementations in healthcare in LMICs.
Although we attempted to perform a broad search of studies of AI deployed in healthcare in LMICs when performing our database search, we may have missed important papers that would have met our inclusion criteria. We mitigated the risk of this by also performing Google Scholar search with broad search terms, as well as exploring grey literature extensively, looking at papers cited in multiple reviews of AI in healthcare and research presented at various AI and healthcare conferences. Only articles published in English were included. This is a limitation of the review since China is an area with a highly active AI research field. However, there are research articles published in English, produced in China, that we were able to include in the review. Articles also had to have been peer-reviewed, which notably excluded a small number of recently published manuscripts on https://arxiv.org/. We concentrated exclusively on completed studies, which may have resulted in a significant reduction in the number of papers, leaving out ongoing research activity that may have been communicated via other channels. The field of AI research is rapidly evolving, therefore our review has also excluded relevant new research that has been published between the time of our database search and the publishing of this paper.
This systematic review has identified ten articles where a wide variety of AI technologies that have been implemented in varying healthcare settings across seven LMICs. AI has a demonstrated potential in triage, diagnostics and treatment planning settings. However, many challenges and barriers to successful implementation exist. Greater transparency and availability of algorithms and datasets used to train AIs could allow for a great understanding of why particular tools perform well or poorly. Further studies of AI use-cases in healthcare settings are required along a number of avenues, including: prospective studies that demonstrate real-world reliability and cost-effectiveness of AI tools, analyses of end-user perspectives of AI usability and trust in AI technologies, and how to effectively integrate AI systems into existing healthcare infrastructure.
Methods
To identify and map all relevant AI studies in LMICs that addressed our research questions, we considered a systematic scoping review as the most suitable methodology for our evidence review54. We followed five iterative stages as described by Arksey and O’Malley and systematically reviewed identified literature in line with published scoping review guidelines55,56,57. We report our findings in accordance with the Preferred Reporting Items for System Reviews and Meta-Analyses Extension for Scoping Reviews (PRISMA-ScR)58.
Databases searched
Our literature search included five electronic databases: Scopus, EMBASE, MEDLINE, Global Health and APA PsycInfo. A search strategy for each database was developed to identify relevant studies (see Supplementary Table 1 for search terms used). We further expanded our search to include grey literature via Google Scholar59. We also conducted a handsearch of journals and conference papers discussing AI applications in global health.
Overall, we included only peer-reviewed literature. Since the field of AI in healthcare is a rapidly evolving field, numerous publications were available ahead of print. In these instances, we only included pre-prints that had already undergone at least initial peer-review. We also reviewed papers presented at AI conferences, as it is common in the field of AI that publications are made available at key conferences which also peer-review submissions.
Search criteria
We applied a variety of search terms consisting of concepts related to AI, healthcare, and LMICs to identify a broad range of peer-reviewed, original records on AI, health and healthcare in LMICs. Our literature search included records published between 1st January 2009 and 30th September 2021. We limited our search to literature published after 2009, as this year marks the point at which graphic processing units (GPUs) were repurposed for AI applications, thus providing a substantial boost in the speed at which AI models could be trained and implemented60. LMICs were defined based on the World Bank Group Classification of Economies as of January 202161. We only included records describing original studies. Records without full-text and articles such as commentaries, letters, policy briefs and study protocols were excluded. Our search further included records that described a quantitative and/or qualitative evaluation of an implemented AI application related to healthcare. Hence, studies merely describing theoretical AI approaches, such as machine learning methods in a non-specific or non-LMIC context without defining a real-world application of AI in a LMIC health context, were not considered.
Study screening and selection
Records identified by the above database searches were entered into the Covidence Systematic Review Software for further title and abstract review62. Inclusion and exclusion criteria were identified following the PICOS (population, intervention, comparison, outcome, study design) framework (see Table 6 for details)63. Three reviewers (T.C.H., R.S. and M.A.) screened titles and abstracts independently to select those articles fully meeting set inclusion criteria related to the application of AI in healthcare in an LMIC. Discrepancies in reviewer ratings were discussed and decided within the entire research team (T.C.H., R.S., M.A., St.B. and S.B.). Once relevant articles had been identified, the reviewers (T.C.H., R.S. and M.A.) screened all full texts to exclude those articles which did not meet inclusion based on full-text review.
Data extraction and synthesis
We used a data extraction form to chart characteristics and map key findings from the final set of articles (see Supplementary Fig. 1). Key AI characteristics included aspects such as the application field and context, dataset sources and algorithms used. Additionally, we mapped the specific use of each AI application as an assistant for either patients, health workers, or physicians64. We extracted descriptive and methodological characteristics of each reviewed study. Content mapping focused on extracting and comparing as well as pertinent outcomes and reported lessons learned.
Reporting summary
Further information on research design is available in the Nature Research Reporting Summary linked to this article.
Data availability
All data generated and analysed during this study are included in the article and its supplementary information files.
References
Atkinson, R. D. & Castro, D. Digital Quality of Life: Understanding the Personal and Social Benefits of the Information Technology Revolution. https://papers.ssrn.com/abstract=1278185 (2008).
Murdoch, T. B. & Detsky, A. S. The inevitable application of big data to health care. JAMA 309, 1351–1352 (2013).
Topol, E. The Creative Destruction Of Medicine: How The Digital Revolution Will Create Better Health Care (Basic Books, 2012).
Ceruzzi, P. E. Computing: A Concise History. (MIT Press, 2012).
Wang, P. On defining artificial intelligence. J. Artif. Gen. Intell. 10, 1–37 (2019).
Russell, S. & Norvig, P. Artificial Intelligence: A Modern Approach (Prentice Hall, 2002).
Davenport, T. & Kalakota, R. The potential for artificial intelligence in healthcare. Future Healthc. J. 6, 94–98 (2019).
Liang, H. et al. Evaluation and accurate diagnoses of pediatric diseases using artificial intelligence. Nat. Med. 25, 433–438 (2019).
Steinhubl, S. R., Muse, E. D. & Topol, E. J. The emerging field of mobile health. Sci. Transl. Med. 7, 283rv3 (2015).
Goldhahn, J., Rampton, V. & Spinas, G. A. Could artificial intelligence make doctors obsolete? BMJ 363, k4563 (2018).
Reddy, C. L., Mitra, S., Meara, J. G., Atun, R. & Afshar, S. Artificial Intelligence and its role in surgical care in low-income and middle-income countries. Lancet Digit. Health 1, e384–e386 (2019).
Frenk, J. et al. Health professionals for a new century: transforming education to strengthen health systems in an interdependent world. Lancet 376, 1923–1958 (2010).
Oren, O., Gersh, B. J. & Bhatt, D. L. Artificial intelligence in medical imaging: switching from radiographic pathological data to clinically meaningful endpoints. Lancet Digit. Health 2, e486–e488 (2020).
Lee, J. et al. Interventions to improve district-level routine health data in low-income and middle-income countries: a systematic review. BMJ Glob. Health 6, e004223 (2021).
Ugarte-Gil, C. et al. Implementing a socio-technical system for computer-aided tuberculosis diagnosis in Peru: A field trial among health professionals in resource-constraint settings. Health Inform. J. 26, 2762–2775 (2020).
Ganju, A., Satyan, S., Tanna, V. & Menezes, S. R. AI for improving children’s health: a community case study. Front. Artif. Intell. 3, 544972 (2021).
Love, S. M. et al. Palpable breast lump triage by minimally trained operators in mexico using computer-assisted diagnosis and low-cost ultrasound. J. Glob. Oncol. https://doi.org/10.1200/JGO.17.00222 (2018).
Garzon-Chavez, D. et al. Adapting for the COVID-19 pandemic in Ecuador, a characterization of hospital strategies and patients. PLoS ONE 16, e0251295 (2021).
MacPherson, P. et al. Computer-aided X-ray screening for tuberculosis and HIV testing among adults with cough in Malawi (the PROSPECT study): A randomised trial and cost-effectiveness analysis. PLoS Med. 18, e1003752 (2021).
Zhou, N. et al. Concordance study between IBM watson for oncology and clinical practice for patients with cancer in China. Oncologist 24, 812–819 (2019).
Kisling, K. et al. Fully automatic treatment planning for external-beam radiation therapy of locally advanced cervical cancer: a tool for low-resource clinics. J. Glob. Oncol. https://doi.org/10.1200/JGO.18.00107 (2019).
Wang, D. et al. “Brilliant AI Doctor” in rural clinics: challenges in AI-powered clinical decision support system deployment. in Proceedings of the 2021 CHI Conference on Human Factors in Computing Systems 1–18 (ACM, 2021).
Fan, X. et al. Utilization of self-diagnosis health chatbots in real-world settings: case study. J. Med. Internet Res. 23, e19928 (2021).
Wang, L. et al. CASS: towards building a social-support chatbot for online health community. Proc. ACM Hum.-Comput. Interact. 5, 1-31 (2021).
Bumrungrad International Hospital. IBM Watson for Oncology Demo. https://www.youtube.com/watch?v=338CIHlVi7A (2015).
Guo, Y., Hao, Z., Zhao, S., Gong, J. & Yang, F. Artificial intelligence in health care: bibliometric analysis. J. Med. Internet Res. 22, e18228 (2020).
Lu, W. et al. Applications of artificial intelligence in ophthalmology: general overview. J. Ophthalmol. 2018, 5278196 (2018).
Amisha, Malik, P., Pathania, M. & Rathaur, V. K. Overview of artificial intelligence in medicine. J. Fam. Med. Prim. Care 8, 2328–2331 (2019).
Roberts, M. et al. Common pitfalls and recommendations for using machine learning to detect and prognosticate for COVID-19 using chest radiographs and CT scans. Nat. Mach. Intell. 3, 199–217 (2021).
Kelly, C. J., Karthikesalingam, A., Suleyman, M., Corrado, G. & King, D. Key challenges for delivering clinical impact with artificial intelligence. BMC Med. 17, 195 (2019).
Mathenge, W. et al. Impact of artificial intelligence assessment of diabetic retinopathy on referral service uptake in a low resource setting: The RAIDERS randomized trial. Ophthalmol. Sci. 2, 100168 (2022).
Ruamviboonsuk, P. et al. Real-time diabetic retinopathy screening by deep learning in a multisite national screening programme: a prospective interventional cohort study. Lancet Digit. Health 4, e235–e244 (2022).
Mhlanga, M., Cimini, T., Amaechi, M., Nwaogwugwu, C. & McGahan, A. From A to O-Positive: Blood Delivery Via Drones in Rwanda. Reach Alliance https://reachalliance.org/wp-content/uploads/2021/03/Zipline-Rwanda-Final-April19.pdf (2021).
Liu, X. et al. A comparison of deep learning performance against health-care professionals in detecting diseases from medical imaging: a systematic review and meta-analysis. Lancet Digit. Health 1, e271–e297 (2019).
Nagendran, M. et al. Artificial intelligence versus clinicians: systematic review of design, reporting standards, and claims of deep learning studies. BMJ 368, m689 (2020).
Žliobaitė, I., Pechenizkiy, M. & Gama, J. An overview of concept drift applications. in Big Data Analysis: New Algorithms for a New Society (eds. Japkowicz, N. & Stefanowski, J.) 91–114 (Springer International Publishing, 2016).
5 Ways to Deal with the Lack of Data in Machine Learning. KDnuggets. https://www.kdnuggets.com/5-ways-to-deal-with-the-lack-of-data-in-machine-learning.html/.
GIZ. From Strategy To Implementation - On The Pathways Of The Youngest Countries In Sub-saharan Africa Towards Digital Transformation Of Health Systems. https://www.governinghealthfutures2030.org/pdf/resources/FromStrategyToImplementation-GIZReport.pdf (2021).
Nutley, T. & Reynolds, H. Improving the use of health data for health system strengthening. Glob. Health Action 6, 20001 (2013).
Ye, Y., Wamukoya, M., Ezeh, A., Emina, J. B. O. & Sankoh, O. Health and demographic surveillance systems: a step towards full civil registration and vital statistics system in sub-Sahara Africa? BMC Public Health 12, 741 (2012).
Coiera, E. The last mile: where artificial intelligence meets reality. J. Med. Internet Res. 21, e16323 (2019).
Cabitza, F., Campagner, A. & Balsano, C. Bridging the “last mile” gap between AI implementation and operation: “data awareness” that matters. Ann. Transl. Med. 8, 501 (2020).
Asan, O. & Choudhury, A. Research trends in artificial intelligence applications in human factors health care: mapping review. JMIR Hum. Factors 8, e28236 (2021).
Wallis, L., Blessing, P., Dalwai, M. & Shin, S. D. Integrating mHealth at point of care in low- and middle-income settings: the system perspective. Glob. Health Action 10, 1327686 (2017).
Hengstler, M., Enkel, E. & Duelli, S. Applied artificial intelligence and trust—the case of autonomous vehicles and medical assistance devices. Technol. Forecast. Soc. Change 105, 105–120 (2016).
Nundy, S., Montgomery, T. & Wachter, R. M. Promoting trust between patients and physicians in the era of artificial intelligence. JAMA 322, 497–498 (2019).
Gafni, R. & Pavel, T. Cyberattacks against the health-care sectors during the COVID-19 pandemic. Inf. Comput. Secur. 30, 137–150 (2021).
Venkatesh, V. & Bala, H. Technology acceptance model 3 and a research agenda on interventions. Decis. Sci. 39, 273–315 (2008).
Wolff, J., Pauling, J., Keck, A. & Baumbach, J. The economic impact of artificial intelligence in health care: systematic review. J. Med. Internet Res. 22, e16866 (2020).
Sanyal, C., Stolee, P., Juzwishin, D. & Husereau, D. Economic evaluations of eHealth technologies: a systematic review. PLoS ONE 13, e0198112 (2018).
Chawla, S. et al. Electricity and generator availability in LMIC hospitals: improving access to safe surgery. J. Surg. Res. 223, 136–141 (2018).
Antwi, W. K., Akudjedu, T. N. & Botwe, B. O. Artificial intelligence in medical imaging practice in Africa: a qualitative content analysis study of radiographers’ perspectives. Insights Imaging 12, 80 (2021).
Ng, M. et al. Effective coverage: a metric for monitoring universal health coverage. PLoS Med. 11, e1001730 (2014).
Munn, Z. et al. Systematic review or scoping review? Guidance for authors when choosing between a systematic or scoping review approach. BMC Med. Res. Methodol. 18, 143 (2018).
Arksey, H. & O’Malley, L. Scoping studies: towards a methodological framework. Int. J. Soc. Res. Methodol. 8, 19–32 (2005).
Peters, M. D. J. et al. Guidance for conducting systematic scoping reviews. JBI Evid. Implement. 13, 141–146 (2015).
Muka, T. et al. A 24-step guide on how to design, conduct, and successfully publish a systematic review and meta-analysis in medical research. Eur. J. Epidemiol. 35, 49–60 (2020).
Tricco, A. C. et al. PRISMA extension for scoping reviews (PRISMA-ScR): checklist and explanation. Ann. Intern. Med. 169, 467–473 (2018).
Haddaway, N. R., Collins, A. M., Coughlin, D. & Kirk, S. The role of google scholar in evidence reviews and its applicability to grey literature searching. PLoS ONE 10, e0138237 (2015).
Raina, R., Madhavan, A. & Ng, A. Y. Large-scale deep unsupervised learning using graphics processors. In Proceedings of the 26th Annual International Conference on Machine Learning 873–880 (Association for Computing Machinery, 2009).
World Bank Country and Lending Groups – World Bank Data Help Desk. https://datahelpdesk.worldbank.org/knowledgebase/articles/906519-world-bank-country-and-lending-groups.
Harrison, H., Griffin, S. J., Kuhn, I. & Usher-Smith, J. A. Software tools to support title and abstract screening for systematic reviews in healthcare: an evaluation. BMC Med. Res. Methodol. 20, 7 (2020).
Methley, A. M., Campbell, S., Chew-Graham, C., McNally, R. & Cheraghi-Sohi, S. PICO, PICOS and SPIDER: a comparison study of specificity and sensitivity in three search tools for qualitative systematic reviews. BMC Health Serv. Res. 14, 579 (2014).
Artificial Intelligence in Global Health: Defining a Collective Path Forward. https://www.usaid.gov/sites/default/files/documents/1864/AI-in-Global-Health_webFinal_508.pdf (2019).
Bereskin, Caulder, P. L.-I., Kovarik, R. & Cowan, C. AI in focus: BlueDot and the response to COVID-19. Lexology https://www.lexology.com/library/detail.aspx?g=a94f63b4-2829-4f62-97f7-43f2aecd12a6 (2020).
ASEAN BioDiaspora Virtual Center. COVID-19 Situational Report in the ASEAN Region. 16 https://asean.org/wp-content/uploads/2021/10/COVID-19_Situational-Report_ASEAN-BioDiaspora-Regional-Virtual-Center_11Oct2021-1.pdf (2021).
Smart delivery robot-Pudu robotics. Smart delivery robot-Pudu robotics https://www.pudutech.com/.
Simonite, T. Chinese Hospitals Deploy AI to Help Diagnose Covid-19. Wired.
Li, K. et al. Assessing the predictive accuracy of lung cancer, metastases, and benign lesions using an artificial intelligence-driven computer aided diagnosis system. Quant. Imaging Med. Surg. 11, 3629–3642 (2021).
Weinstein, E. China’s Use of AI in its COVID-19 Response. https://cset.georgetown.edu/publication/chinas-use-of-ai-in-its-covid-19-response/ (2020).
Liu, X. et al. A 2-year investigation of the impact of the computed tomography–derived fractional flow reserve calculated using a deep learning algorithm on routine decision-making for coronary artery disease management. Eur. Radiol. 31, 7039–7046 (2021).
Ruijin Hospital: Develop AI-powered chronic disease management products with 4Paradigm. 4Paradigm. https://en.4paradigm.com/content/details_262_1198.html.
Han, M. Langfang’s epidemic prevention and control strategy, robots are online on duty. Beijing Daily https://ie.bjd.com.cn/5b165687a010550e5ddc0e6a/contentApp/5b1a1310e4b03aa54d764015/AP5e4aae66e4b0c4aab142c4d8?isshare=1&app=8ED108F8-A848-43A8-B32F-83FD7330B638&from=timeline (2020).
Research on the Application of Intelligent Triage Innovation Technology in Southwest Medical University Hospital. Futong. http://www.futong.com.cn/intell-medical-case2.html (2020).
iFLYTEK Corporate Social Responsibility Report. https://www.iflytek.com/en/usr/uploads/2020/09/csr.pdf (2020).
Across China: Drones for blood deliveries take off in China - Xinhua | English.news.cn. http://www.xinhuanet.com/english/2021-03/27/c_139839745.htm (2021).
Truog, S., Lawrence, E., Defawe, O., Ramirez Rufino, S. & Perez Richiez, O. Medical Cargo Drones in Rural Dominican Republic. https://publications.iadb.org/publications/english/document/Medical-Cargo-Drones-in-Rural-Dominican-Republic.pdf (2020).
Knoblauch, A. M. et al. Bi-directional drones to strengthen healthcare provision: experiences and lessons from Madagascar, Malawi and Senegal. BMJ Glob. Health 4, e001541 (2019).
He, J. et al. Artificial intelligence-based screening for diabetic retinopathy at community hospital. Eye 34, 572–576 (2020).
Rajalakshmi, R., Subashini, R., Anjana, R. M. & Mohan, V. Automated diabetic retinopathy detection in smartphone-based fundus photography using artificial intelligence. Eye 32, 1138–1144 (2018).
Mollura, D. J. et al. Artificial intelligence in low- and middle-income countries: innovating global health radiology. Radiology 297, 513–520 (2020).
Partnerships. Alexapath. http://alexapath.com/Company/Partnership (2020).
Nakasi, R., Tusubira, J. F., Zawedde, A., Mansourian, A. & Mwebaze, E. A web-based intelligence platform for diagnosis of malaria in thick blood smear images: a case for a developing country. In 2020 IEEE/CVF Conference on Computer Vision and Pattern Recognition Workshops (CVPRW) 4238–4244 (IEEE, 2020).
Morales, H. M. P., Guedes, M., Silva, J. S. & Massuda, A. COVID-19 in Brazil—preliminary analysis of response supported by artificial intelligence in municipalities. Front. Digit. Health 3, 52 (2021).
Chinas AI doctor-bot help each doctor treat 600-700 patients daily. China Experience. https://www.china-experience.com/china-experience-insights/chinas-ai-doctor-bot-help-each-doctor-treat-600-700-patients-daily (2020).
Sapio Analytics launches ‘empathetic’ healthcare chatbot. MobiHealthNews https://www.mobihealthnews.com/news/asia/sapio-analytics-launches-empathetic-healthcare-chatbot (2021).
Index Labs TZ Company Limited. eShangazi is one-year-old! Medium https://medium.com/@indexlabstz/eshangazi-is-one-year-old-46b2b93978a4 (2018).
Patient Retention Solution. BroadReach Healthcare https://broadreachcorporation.com/patient-retention-solution/ (2020).
Sophisticated nudging in HIV: combining predictive analytics and behavioural insights. Indlela https://indlela.org/sophisticated-nudging-in-hiv-combining-predictive-analytics-and-behavioural-insights/ (2021).
Digital health: 5 innovative projects. Terre des hommes. https://www.tdh.ch/en/news/digital-health-5-innovative-projects (2021).
Ubenwa - 2019 In Review. https://www.ubenwa.ai/ubenwa-2019-highlight.html (2020).
Acknowledgements
We thank Yash Kulkarni (Imperial College London) and Nicholas Robinson (Imperial College London) for their assistance in our initial database search. We also thank Dr. Laurence Court (MD Anderson Cancer Centre) for having insightful discussions with us about automated radiation treatment planning and their research. The authors wish to thank the German Research Foundation (Deutsche Forschungsgemeinschaft) for supporting this work as part of a Deutsche Forschungsgemeinschaft–funded research unit (Forschungsgruppe FOR 2936/project: 409670289). For the publication fee we acknowledge financial support by Deutsche Forschungsgemeinschaft within the funding programme “Open Access Publikationskosten“ as well as by Heidelberg University.
Author information
Authors and Affiliations
Contributions
The authors confirm contribution to the paper as follows: conceptualisation: T.C.H., R.S., St.B. and S.B.; data curation: T.C.H. and R.S.; formal analysis: T.C.H., St.B. and S.B.; funding acquisition: N.A.; investigation: T.C.H., R.S., M.A., St.B. and S.B.; methodology: T.C.H., R.S., St.B. and S.B.; project administration: T.C.H.; resources: T.C.H. and R.S.; software: not applicable; supervision: T.C.H., St.B. and S.B.; validation: T.C.H., R.S. and M.A.; visualisation: T.C.H. and St.B.; writing – original draft: T.C.H., R.S., M.A. and S.B.; and writing – review & editing: T.C.H., R.S., M.A., St.B. and S.B. All authors reviewed the results and approved the final version of the manuscript.
Corresponding author
Ethics declarations
Competing interests
The authors declare no competing interests.
Additional information
Publisher’s note Springer Nature remains neutral with regard to jurisdictional claims in published maps and institutional affiliations.
Supplementary information
Rights and permissions
Open Access This article is licensed under a Creative Commons Attribution 4.0 International License, which permits use, sharing, adaptation, distribution and reproduction in any medium or format, as long as you give appropriate credit to the original author(s) and the source, provide a link to the Creative Commons license, and indicate if changes were made. The images or other third party material in this article are included in the article’s Creative Commons license, unless indicated otherwise in a credit line to the material. If material is not included in the article’s Creative Commons license and your intended use is not permitted by statutory regulation or exceeds the permitted use, you will need to obtain permission directly from the copyright holder. To view a copy of this license, visit http://creativecommons.org/licenses/by/4.0/.
About this article
Cite this article
Ciecierski-Holmes, T., Singh, R., Axt, M. et al. Artificial intelligence for strengthening healthcare systems in low- and middle-income countries: a systematic scoping review. npj Digit. Med. 5, 162 (2022). https://doi.org/10.1038/s41746-022-00700-y
Received:
Accepted:
Published:
DOI: https://doi.org/10.1038/s41746-022-00700-y
This article is cited by
-
Research and application of artificial intelligence in dentistry from lower-middle income countries – a scoping review
BMC Oral Health (2024)
-
Artificial Intelligence in Chest Radiology: Advancements and Applications for Improved Global Health Outcomes
Current Pulmonology Reports (2024)
-
Harnessing oncology real-world data with AI
Nature Cancer (2023)
-
A transparent artificial intelligence framework to assess lung disease in pulmonary hypertension
Scientific Reports (2023)
-
Feasibility and accuracy of the screening for diabetic retinopathy using a fundus camera and an artificial intelligence pre-evaluation application
Acta Diabetologica (2023)